Note
Go to the end to download the full example code.
Relating Gender and IQ¶
Going back to the brain size + IQ data, test if the VIQ of male and female are different after removing the effect of brain size, height and weight.
Notice that here ‘Gender’ is a categorical value. As it is a non-float data type, statsmodels is able to automatically infer this.
import pandas
from statsmodels.formula.api import ols
data = pandas.read_csv("../brain_size.csv", sep=";", na_values=".")
model = ols("VIQ ~ Gender + MRI_Count + Height", data).fit()
print(model.summary())
# Here, we don't need to define a contrast, as we are testing a single
# coefficient of our model, and not a combination of coefficients.
# However, defining a contrast, which would then be a 'unit contrast',
# will give us the same results
print(model.f_test([0, 1, 0, 0]))
OLS Regression Results
==============================================================================
Dep. Variable: VIQ R-squared: 0.246
Model: OLS Adj. R-squared: 0.181
Method: Least Squares F-statistic: 3.809
Date: Tue, 16 Jul 2024 Prob (F-statistic): 0.0184
Time: 11:56:55 Log-Likelihood: -172.34
No. Observations: 39 AIC: 352.7
Df Residuals: 35 BIC: 359.3
Df Model: 3
Covariance Type: nonrobust
==================================================================================
coef std err t P>|t| [0.025 0.975]
----------------------------------------------------------------------------------
Intercept 166.6258 88.824 1.876 0.069 -13.696 346.948
Gender[T.Male] 8.8524 10.710 0.827 0.414 -12.890 30.595
MRI_Count 0.0002 6.46e-05 2.615 0.013 3.78e-05 0.000
Height -3.0837 1.276 -2.417 0.021 -5.674 -0.494
==============================================================================
Omnibus: 7.373 Durbin-Watson: 2.109
Prob(Omnibus): 0.025 Jarque-Bera (JB): 2.252
Skew: 0.005 Prob(JB): 0.324
Kurtosis: 1.823 Cond. No. 2.40e+07
==============================================================================
Notes:
[1] Standard Errors assume that the covariance matrix of the errors is correctly specified.
[2] The condition number is large, 2.4e+07. This might indicate that there are
strong multicollinearity or other numerical problems.
<F test: F=0.683196084584229, p=0.4140878441244694, df_denom=35, df_num=1>
Here we plot a scatter matrix to get intuitions on our results. This goes beyond what was asked in the exercise
# This plotting is useful to get an intuitions on the relationships between
# our different variables
from pandas import plotting
import matplotlib.pyplot as plt
# Fill in the missing values for Height for plotting
data["Height"].fillna(method="pad", inplace=True)
# The parameter 'c' is passed to plt.scatter and will control the color
# The same holds for parameters 'marker', 'alpha' and 'cmap', that
# control respectively the type of marker used, their transparency and
# the colormap
plotting.scatter_matrix(
data[["VIQ", "MRI_Count", "Height"]],
c=(data["Gender"] == "Female"),
marker="o",
alpha=1,
cmap="winter",
)
fig = plt.gcf()
fig.suptitle("blue: male, green: female", size=13)
plt.show()
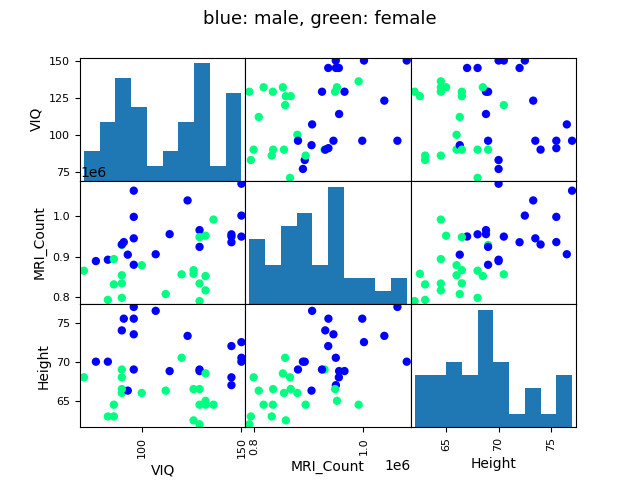
/home/runner/work/scientific-python-lectures/scientific-python-lectures/packages/statistics/examples/solutions/plot_brain_size.py:39: FutureWarning: A value is trying to be set on a copy of a DataFrame or Series through chained assignment using an inplace method.
The behavior will change in pandas 3.0. This inplace method will never work because the intermediate object on which we are setting values always behaves as a copy.
For example, when doing 'df[col].method(value, inplace=True)', try using 'df.method({col: value}, inplace=True)' or df[col] = df[col].method(value) instead, to perform the operation inplace on the original object.
data["Height"].fillna(method="pad", inplace=True)
/home/runner/work/scientific-python-lectures/scientific-python-lectures/packages/statistics/examples/solutions/plot_brain_size.py:39: FutureWarning: Series.fillna with 'method' is deprecated and will raise in a future version. Use obj.ffill() or obj.bfill() instead.
data["Height"].fillna(method="pad", inplace=True)
Total running time of the script: (0 minutes 0.222 seconds)