Note
Go to the end to download the full example code.
3.1.6.5. Multiple Regression¶
Calculate using ‘statsmodels’ just the best fit, or all the corresponding statistical parameters.
Also shows how to make 3d plots.
# Original author: Thomas Haslwanter
import numpy as np
import matplotlib.pyplot as plt
import pandas
# For 3d plots. This import is necessary to have 3D plotting below
from mpl_toolkits.mplot3d import Axes3D
# For statistics. Requires statsmodels 5.0 or more
from statsmodels.formula.api import ols
# Analysis of Variance (ANOVA) on linear models
from statsmodels.stats.anova import anova_lm
Generate and show the data
x = np.linspace(-5, 5, 21)
# We generate a 2D grid
X, Y = np.meshgrid(x, x)
# To get reproducible values, provide a seed value
rng = np.random.default_rng(27446968)
# Z is the elevation of this 2D grid
Z = -5 + 3 * X - 0.5 * Y + 8 * np.random.normal(size=X.shape)
# Plot the data
ax: Axes3D = plt.figure().add_subplot(projection="3d")
surf = ax.plot_surface(X, Y, Z, cmap="coolwarm", rstride=1, cstride=1)
ax.view_init(20, -120)
ax.set_xlabel("X")
ax.set_ylabel("Y")
ax.set_zlabel("Z")
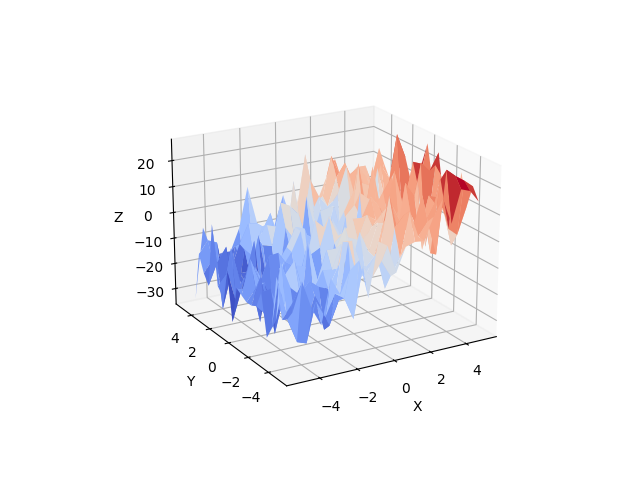
Text(-0.10764513121260137, 0.009865032686848034, 'Z')
Multilinear regression model, calculating fit, P-values, confidence intervals etc.
# Convert the data into a Pandas DataFrame to use the formulas framework
# in statsmodels
# First we need to flatten the data: it's 2D layout is not relevant.
X = X.flatten()
Y = Y.flatten()
Z = Z.flatten()
data = pandas.DataFrame({"x": X, "y": Y, "z": Z})
# Fit the model
model = ols("z ~ x + y", data).fit()
# Print the summary
print(model.summary())
print("\nRetrieving manually the parameter estimates:")
print(model._results.params)
# should be array([-4.99754526, 3.00250049, -0.50514907])
# Perform analysis of variance on fitted linear model
anova_results = anova_lm(model)
print("\nANOVA results")
print(anova_results)
plt.show()
OLS Regression Results
==============================================================================
Dep. Variable: z R-squared: 0.579
Model: OLS Adj. R-squared: 0.577
Method: Least Squares F-statistic: 300.7
Date: Wed, 19 Feb 2025 Prob (F-statistic): 6.43e-83
Time: 17:24:18 Log-Likelihood: -1552.0
No. Observations: 441 AIC: 3110.
Df Residuals: 438 BIC: 3122.
Df Model: 2
Covariance Type: nonrobust
==============================================================================
coef std err t P>|t| [0.025 0.975]
------------------------------------------------------------------------------
Intercept -4.4332 0.390 -11.358 0.000 -5.200 -3.666
x 3.0861 0.129 23.940 0.000 2.833 3.340
y -0.6856 0.129 -5.318 0.000 -0.939 -0.432
==============================================================================
Omnibus: 0.560 Durbin-Watson: 1.967
Prob(Omnibus): 0.756 Jarque-Bera (JB): 0.651
Skew: -0.077 Prob(JB): 0.722
Kurtosis: 2.893 Cond. No. 3.03
==============================================================================
Notes:
[1] Standard Errors assume that the covariance matrix of the errors is correctly specified.
Retrieving manually the parameter estimates:
[-4.43322435 3.08614608 -0.68556194]
ANOVA results
df sum_sq mean_sq F PR(>F)
x 1.0 38501.973182 38501.973182 573.111646 1.365553e-81
y 1.0 1899.955512 1899.955512 28.281320 1.676135e-07
Residual 438.0 29425.094352 67.180581 NaN NaN
Total running time of the script: (0 minutes 0.110 seconds)